This website uses cookies so that we can provide you with the best user experience possible. Cookie information is stored in your browser and performs functions such as recognising you when you return to our website and helping our team to understand which sections of the website you find most interesting and useful.
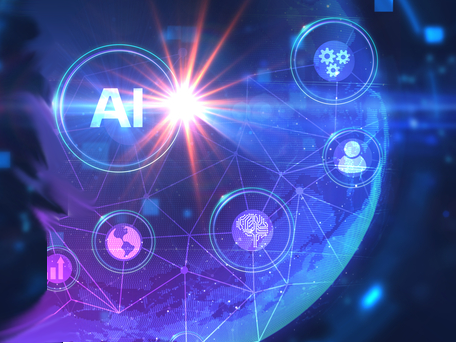
Image recognition is a rapidly evolving technology that uses artificial intelligence tools like computer vision and machine learning to identify digital images. In order to do this, the images are transformed into descriptions that are used to convey meaning. A simple explanation of how it works is that image examples and definitions (said definitions set by humans) are given to the computer, and then the computer is provided with more image examples to identify in repeating tests until the computer learns to correctly identify the images being presented.
Facial Recognition
A great deal of funding and development is dedicated to facial recognition software. From Facebook suggesting tags for your friends and family in photos to iPhone 8’s facial ID functionality, to use in the criminal justice system, this technology has been developed quite extensively. Facial recognition has come a long way, and while still prone to errors, it can often be extremely accurate about identifying individuals, as well as their mood and facial expressions.
Image recognition is also used widely for sales and marketing. EBay has an Image Search function that searches for eBay items from an uploaded photo. Some marketers are using photos uploaded to social media in combination with hashtags and locations to identify people, where they are, what they are eating and drinking, and even sometimes what they are wearing. They use this information to target ads.
Image recognition will factor into self-driving cars. Artificial intelligence is being taught to identify objects in the road, other vehicles, and pedestrians. It is also being used for augmented reality applications and games.
Image Recognition for the Blind
Image recognition has also been incorporated into a number of applications to help people who are blind or who have low vision to know what is depicted in digital photos and to identify objects viewed in person. Some of these applications work in conjunction with a smartphone, some are adjunct plug-ins to existing programs and platforms. Orcam MyEye, Seeing AI by Microsoft, TappTappSee, and Aipoly Vision are all being used to identify objects. Some of these applications can read text and even handwriting. Google Chrome has recently released a plug-in that works with computer screen readers such as NVDA or JAWS to identify objects in photos found on a computer screen.
How Good is It?
But how good and usable is this image recognition technology? Can it replace human-generated alternative text (alt-text) to identifying images for those who can’t see them? As an experiment, we tested the Google Chrome plug-in Google Lens for its image recognition.
This was by no means an exhaustive test, but here are some of the more interesting results:
Some were surprisingly accurate: “Image may contain grass dog”
“Image may contain horse outdoors”
Others were accurate, but left out important information, like this one, showing a soccer team: “Image may contain 21 people smiling outdoors”
This one, which is clearly a birthday cake: “Image may contain food.”
Or this one, which any sighted person would instantly recognize as a jack-o-lantern: “Image may contain night and fire.” No mention of a pumpkin at all.
Or this one, of several deer: “Image may contain tree plant outdoor dog nature.” Nope. Not dogs.
Or the worst identification, three terrifying Halloween-style clowns, identified as “image may contain three people smiling.” Technically, two of these clowns are smiling, but that is not the idea meant to be conveyed by any of these creepy clown pictures.
We looked at an entire page of baseball player images. Most simply identified as a person outdoors or, person playing sports. Only this image was correctly identified as “image may contain one or more people, people playing sports baseball.”
The takeaway here is that while the image recognition by artificial intelligence is in some cases shockingly accurate, and surprisingly useful, in many cases the intent of the photo is completely lost.
Why are the Results so Erratic?
Here’s why the accuracy is so variable: image recognition relies entirely on a database of human-defined images to identify what the computer is “seeing.” The better the definitions, the more accurate the identification. Databases that have been more developed result in more accuracy. An artificial intelligence tool for image recognition can only identify what it has been told. And each has its own database of image definitions. Some can identify a “smile” and “people” and can count them, but can’t always tell if there are 21 people as part of a soccer team or 21 people at a family picnic. Some have databases that are trained to identify plants, or birds, or insects. (How many of us have tried out the “identify this” apps for gardening or wildlife?)
The human brain can store and recall all the nuanced details required to correctly identify a photo of 3 scary clowns as more than “3 people smiling,” but unless the computer has been taught how to identify a clown (and all 3 of these look utterly different), the technology just doesn’t know what it is “seeing.” The database identifying deer as “dogs” may simply not have any definitions of “deer.”
When and How Does it Get Better?
You may be thinking that surely in time, the databases will become more full of image definitions and the accuracy will improve, in much the same way crowd-sourcing improved Google Maps. While this may be true, the larger the database of image definitions, the longer it will take to identify what those images are. Most image recognition software runs on a special Graphics Processing Unit (GPU) which will run several cores simultaneously allowing for thousands of operations to take place at a time. That said, there is still a limit to how much data can be run through a GPU at a time which limits how many definitions it can parse. For now, only very limited definitions of objects exist in most image recognition databases. The purpose of the various image databases will inform the kinds of definitions that they contain. Criminal justice facial recognition software probably doesn’t care that the image may contain a leather coat, or that there is a dog in the photo. Plant identification apps don’t identify insects in the uploaded photos.
Thus, even with image recognition software that is designed for general use (rather than specifics like faces, plants, or birds), we are still going to get results that identify these two cute penguins as “Image may contain one person outdoor.”
For now, humans are vastly superior at image recognition to any computer. This means for accessibility purposes, human-authored text alternatives still need to be used to explain images in digital content. Otherwise, this picture below will be identified as “image may contain a person smiling indoors” rather than the accurate “toddler grinning with both fists raised in triumph after he successfully balances a carrot on every single horizontal cabinet and drawer handle in the kitchen.”
But Wait There’s More…
Even more difficult to identify are images such as charts, graphs, and diagrams. At this time, no technology exists that can accurately describe this image of a bar graph, pie chart, flow chart or diagram:
Here are a few examples:
The image recognition simply identifies this chart as “unknown.” Alternative text is really the only way to define this particular image.
Or this image:
Even if the AI can identify this as a dog, the meaning being conveyed is in the descriptive parts of the harness it is wearing. “Dog wearing harness. Shows the parts of the harness as follows: Chest strap goes across the dog’s chest and features a d-ring in the center front. A connector ring is on each end of the chest strap at the dog’s shoulder. The shoulder strap runs over the dog’s back from each connector ring at the shoulder, and a belly strap runs from the same connector ring under the dog’s chest.”
Sometimes Alt-Text isn’t Best
Some images simply aren’t easy to convey even using text alternatives. It’s extremely impractical for alt text to be used to describe a section of Google maps to someone who cannot see it. The choice there is to provide directions, not a full description of the map. This is something that maps applications do quite well. They also have adjunct features to help nearby restaurants, gas stations, and stores. Even for a sighted person, this is a more useful way to find what is wanted than scanning the entire map to find a coffee shop.
Other images that aren’t best served by alt-text are things like flow charts or org charts. These work best if set up as lists, or hierarchies of text with headings.
This org chart is best conveyed to someone using assistive technology as a nested list:
- Director
- Manager – Project 1
- Analysts
- Architect
- Developer
- Tester
- Manager – Project 2
- Analysts
- Architect
- Developer
- Tester
- Manager – Project 3
- Analysts
- Architect
- Developer
- Tester
- Manager – Project 4
- Analysts
- Architect
- Developer
- Tester
- Manager – Project 1
AI isn’t Ready to Replace Alt Text
Image Recognition by artificial intelligence is making great strides, particularly facial recognition. But as a tool to identify images for people who are blind or have low vision, for the foreseeable future, we are still going to need alt text added to most images found in digital content. Too many images require context to be fully understood.
To learn more about adding alt text to images, check out our other resources or contact Equidox for more information!
Tammy Albee
Tammy Albee | Director of Marketing | Equidox Tammy joined Equidox after four years of experience working at the National Federation of the Blind. She firmly maintains that accessibility is about reaching everyone, regardless of ability, and boosting your market share in the process. "Nobody should be barred from accessing information. It's what drives our modern society."